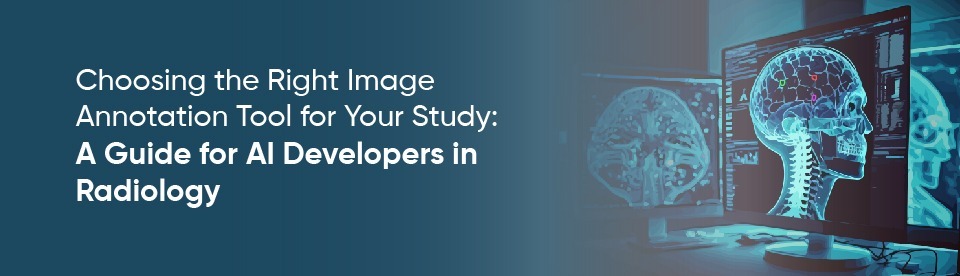
The integration of Artificial intelligence is bringing about a revolution in the field of radiology. An accurate image annotation is crucial for developing robust AI models in radiology. According to a report, the market for healthcare data annotation tools was estimated to be worth USD 129.9 million in 2022, and it is anticipated to grow significantly at a compound annual growth rate (CAGR) of 27.5% between 2023 and 2030. A large number of image annotation tools are available in the market, such as Labellerr, Encord, 3D Slicer, ITK-Snap, Labelbox, SuperAnnotate, etc. Selecting the right tool is pivotal, as it forms a basis for training machine learning (ML) models and improving their ability to analyze and interpret the vast amount of clinical data, leading to surgical guidance and treatment decisions and significantly improving patient outcomes.
Here is a guide for AI developers in radiology to choose the right image annotation tool for your study.
Understand annotation requirements in radiology
Each radiology imaging modality has a unique annotation requisite. The annotation tools should essentially be selected accordingly.
Bounding boxes and polygons
Helpful for locating lesions, tumors, or other abnormalities within images.
Semantic segmentation
Enables precise delineation of organs and pathological regions and for pixel-level classification.
Instance segmentation
Labeling each object in an image with a unique label. For example, labeling each tumor.
Keypoint and landmark annotation
Essential to annotate specific features such as objects that have a small size or for tracking anatomical landmarks, particularly in dynamic studies such as cardiac imaging.
3D Volumetric Annotation
Necessary for annotating structures in three-dimensional space, as seen in CT or MRI scans.
Imaging format compatibility
The tool should support the standard medical imaging formats, including DICOM (Digital Imaging and Communications in Medicine) and TIFF files.
Advanced Visualization
Tools should offer advanced visualization capabilities, including multi-plane views and volume rendering for 3D data. High-precision tools that support fine-grained annotations are crucial for accurately marking small and subtle features.
Collaboration Features
Radiology projects often involve multiple experts. The tools that support collaborative annotation and review should be chosen.
Automated Annotation
AI-assisted tools can accelerate the annotation process by pre-labeling common structures, allowing radiologists to focus on refining annotations. Further, the tools that provide automated error detection can identify and flag potential annotation mistakes, saving time and improving accuracy.
Quality Control Mechanisms
The accuracy of AI models depends heavily on the quality of the annotated data. Tools that offer robust quality control mechanisms be selected. Inter-annotator agreement feature of the tool allows multiple annotators to work on the same images and measure consistency between them. A structured workflow for reviewing and approving annotations ensures that only high-quality data is used for training.
Budget/ pocket friendly
Image annotation tools vary widely in cost, from open-source solutions to premium, subscription-based services. The choice of tool should depend upon the scale of the project and the available budget. Open-source tools can be cost-effective for small to medium-sized projects. Examples include ITK-SNAP and 3D Slicer, which are popular in the medical imaging community. Commercial Tools often provide advanced features, better support, and scalability. Examples include MD.ai and Aiforia, which cater specifically to medical imaging needs.
Data Security and Privacy
Radiology data is highly sensitive, and it’s crucial to choose tools that prioritize data security and privacy and comply with relevant regulations such as HIPAA and other privacy standards to protect patient information from being seen by unauthorized people.
Support and Community
Having access to reliable support and a knowledgeable community can be invaluable, help resolve issues quickly and efficiently. Further, the tools with extensive documentation, tutorials, and FAQs can significantly ease the learning curve.
Trial and Evaluation
Before committing to a particular tool, it is imperative to take the free trials or demo to evaluate the suitability and performance of the tool. It is also necessary to assure that the tool is integrated seamlessly with the existing workflows and software systems.
Conclusion
Choosing the right image annotation tool is a crucial step in developing effective AI models in radiology. By understanding your specific annotation needs, evaluating key features, and considering budget and support into account, always select a tool which guarantees data confidentiality and quality and enhances accuracy and efficiency in your study. Taking the time to trial and evaluate different tools will ensure you make an informed decision that sets your project up for success.