Within the ever-evolving landscape of medical research, the integration of cutting-edge technologies has become indispensable. One such technology making significant strides is that of image annotation. What is medical image annotation? It is a process of labelling and highlighting particular aspects in medical images such as MRIs, CT scans, Mammography, or Ultrasound and X-rays, enables healthcare practitioners make decisions about diagnosis and treatment while laying the foundation for the creation of sophisticated machine learning algorithms. In this blog, we delve into the pivotal role of image annotation and its application in medical research.
Over the years, the concept of medical image annotation has advanced considerably, keeping up with developments in computer science and medical imaging technologies. The development of medical imaging techniques such as MRIs, CT scans, and X-rays in the 1950s produced a vast database of medical images. Initially, radiologists manually assessed these images, labeling and noting areas of interest. During the 1970s-1980s, as computer technology advanced, the first digital medical imaging systems were developed, introducing digital annotations where radiologists could use software to mark and comment on images. Later in the 1980s and 1990s, the idea of Computer-Aided Diagnosis (CAD) surfaced, with the intention of helping radiologists decipher complicated images. CAD systems relied on annotated images to train algorithms to detect abnormalities like tumors and fractures. Today, the field continues to evolve with the development of sophisticated AI models that require high-quality annotated data.
Applications of Medical Image Annotation
1.Precision and Efficiency in Diagnosis and Treatment Planning
Image annotation highlights specific structures and abnormalities that help healthcare providers interpret complex images precisely and efficiently, reducing diagnostic errors and allowing for timely interventions.
2.Training Machine Learning Models
Image annotation is instrumental in training machine learning models to study medical images and recognize patterns, critical features, tumor identification, organ structure evaluation, and anomaly detection.
3.Standardization and Consistency in Medical Imaging Interpretation
Image annotation provides clear and consistent labels for structures and features in medical images, standardizing the interpretation of images across different healthcare providers and institutions. This consistency is important for ensuring uniformity of diagnoses and treatment plans, reducing variability, and improving overall healthcare quality.
4.Supporting Research and Development Initiatives
Image annotation facilitates quantitative analysis of medical images, unlocking a wealth of data for researchers and clinicians. Whether validating hypotheses, developing new algorithms, evaluating the efficacy of novel interventions, refining treatment strategies, or gaining a comprehensive understanding of complex medical conditions, annotated images serve as the bedrock for researchers, providing the requisite foundation to push the boundaries of medical knowledge and practice.
5.Enhancing Medical Education and Training
Annotated medical images serve as invaluable educational resources for medical students, residents, and practicing healthcare professionals. By studying annotated images, learners gain a deeper understanding of anatomy, pathology, and medical imaging techniques. This educational support not only improves diagnostic skills but also contributes to the continuous professional development of healthcare practitioners.
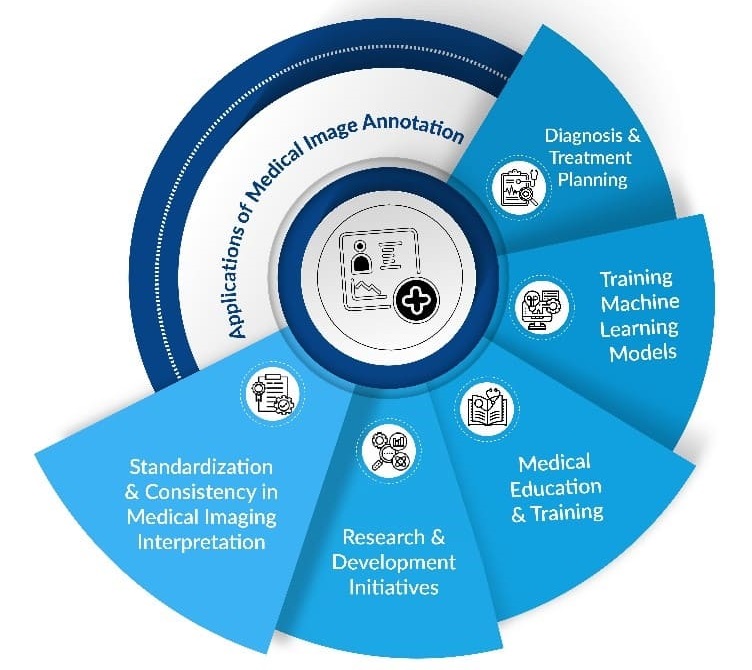
Conclusion
In conclusion, image annotation stands as a linchpin in the realm of medical research, contributing significantly to advancements in technology, diagnostic accuracy, and healthcare practices. From training machine learning models to supporting research initiatives and enhancing medical education, the importance of image annotation in medical research cannot be overemphasized. As we continue to unlock the potential of this technology, the future holds promising prospects for improved patient care and groundbreaking discoveries in the field of medicine.